Could Biases in Artificial Intelligence Databases Present Health Risks to Patients and Financial Risks to Healthcare Providers, including Medical Laboratories?
Clinical laboratories working with AI should be aware of ethical challenges being pointed out by industry experts and legal authorities
Experts are voicing concerns that using artificial intelligence (AI) in healthcare could present ethical challenges that need to be addressed. They say databases and algorithms may introduce bias into the diagnostic process, and that AI may not perform as intended, posing a potential for patient harm.
If true, the issues raised by these experts would have major implications for how clinical laboratories and anatomic pathology groups might use artificial intelligence. For that reason, medical laboratory executives and pathologists should be aware of possible drawbacks to the use of AI and machine-learning algorithms in the diagnostic process.
Is AI Underperforming?
AI’s ability to improve diagnoses, precisely target therapies, and leverage healthcare data is predicted to be a boon to precision medicine and personalized healthcare.
For example, Accenture (NYSE:ACN) says that hospitals will spend $6.6 billion on AI by 2021. This represents an annual growth rate of 40%, according to a report from the Dublin, Ireland-based consulting firm, which states, “when combined, key clinical health AI applications can potentially create $150 billion in annual savings for the United States healthcare economy by 2026.”
But are healthcare providers too quick to adopt AI?
Accenture defines AI as a “constellation of technologies from machine learning to natural language processing that allows machines to sense, comprehend, act, and learn.” However, some experts say AI is not performing as intended, and that it introduces biases in healthcare worthy of investigation.
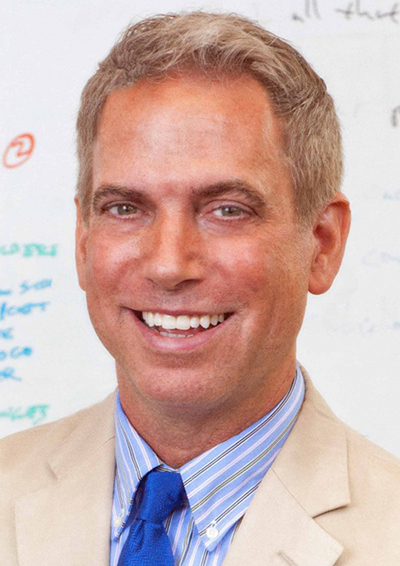
What Goes in Limits What Comes Out
Could machine learning lead to machine decision-making that puts patients at risk? Some legal authorities say yes. Especially when computer algorithms are based on limited data sources and questionable methods, lawyers warn.
Pilar Ossorio PhD, JD, Professor of Law and Bioethics at the University of Wisconsin Law School (UW), toldHealth Data Management (HDM) that genomics databases, such as the Genome-Wide Association Studies (GWAS), house data predominantly about people of Northern European descent, and that could be a problem.
How can AI provide accurate medical insights for people when the information going into databases is limited in the first place? Ossorio pointed to lack of diversity in genomic data. “There are still large groups of people for whom we have almost no genomic data. This is another way in which the datasets that you might use to train your algorithms are going to exclude certain groups of people altogether,” she told HDM.
She also sounded the alarm about making decisions about women’s health when data driving them are based on studies where women have been “under-treated compared with men.”
“This leads to poor treatment, and that’s going to be reflected in essentially all healthcare data that people are using when they train their algorithms,” Ossorio said during a Machine Learning for Healthcare (MLHC) conference covered by HDM.
How Bias Happens
Bias can enter healthcare data in three forms: by humans, by design, and in its usage. That’s according to David Magnus, PhD, Director of the Stanford Center for Biomedical Ethics (SCBE) and Senior Author of a paper published in the New England Journal of Medicine (NEJM) titled, “Implementing Machine Learning in Health Care—Addressing Ethical Challenges.”
The paper’s authors wrote, “Physician-researchers are predicting that familiarity with machine-learning tools for analyzing big data will be a fundamental requirement for the next generation of physicians and that algorithms might soon rival or replace physicians in fields that involve close scrutiny of images, such as radiology and anatomical pathology.”
In a news release, Magnus said, “You can easily imagine that the algorithms being built into the healthcare system might be reflective of different, conflicting interests. What if the algorithm is designed around the goal of making money? What if different treatment decisions about patients are made depending on insurance status or their ability to pay?”
In addition to the possibility of algorithm bias, the authors of the NEJM paper have other concerns about AI affecting healthcare providers:
- “Physicians must adequately understand how algorithms are created, critically assess the source of the data used to create the statistical models designed to predict outcomes, understand how the models function and guard against becoming overly dependent on them.
- “Data gathered about patient health, diagnostics, and outcomes become part of the ‘collective knowledge’ of published literature and information collected by healthcare systems and might be used without regard for clinical experience and the human aspect of patient care.
- “Machine-learning-based clinical guidance may introduce a third-party ‘actor’ into the physician-patient relationship, challenging the dynamics of responsibility in the relationship and the expectation of confidentiality.”
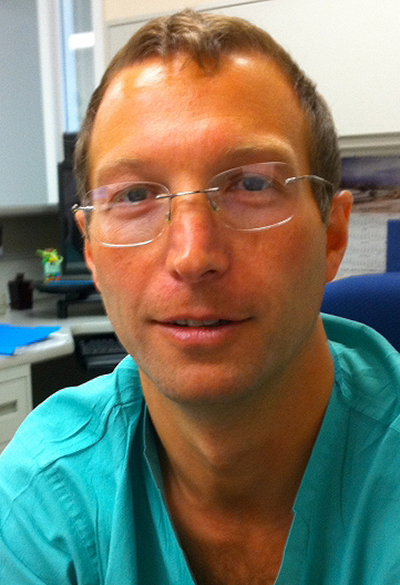
Acknowledge Healthcare’s Differences
Still, the Stanford researchers acknowledge that AI can benefit patients. And that healthcare leaders can learn from other industries, such as car companies, which have test driven AI.
“Artificial intelligence will be pervasive in healthcare in a few years,” said
Nigam Shah, PhD, co-author of the NEJM paper and Associate Professor of Medicine at Stanford, in the news release. He added that healthcare leaders need to be aware of the “pitfalls” that have happened in other industries and be cognizant of data.
“Be careful about knowing the data from which you learn,” he warned.
AI’s ultimate role in healthcare diagnostics is not yet fully known. Nevertheless, it behooves clinical laboratory leaders and anatomic pathologists who are considering using AI to address issues of quality and accuracy of the lab data they are generating. And to be aware of potential biases in the data collection process.
—Donna Marie Pocius
Related Information:
Accenture: Healthcare Artificial Intelligence
Could Artificial Intelligence Do More Harm than Good in Healthcare?
AI Machine Learning Algorithms Are Susceptible to Biased Data
Implementing Machine Learning in Healthcare—Addressing Ethical Challenges
Researchers Say Use of AI in Medicine Raises Ethical Questions