Computer-assisted analysis using Google’s LYNA algorithm shows significant gains in speed required to analyze stained lymph node slides and sensitivity of micrometastases detection in two recent studies
Anatomic pathologists understand the complexities of reviewing slides and samples for signs of cancer’s spread. Two studies involving a new artificial intelligence (AI) algorithm from Google (NASDAQ:GOOGL) claim their “deep learning” LYmph Node Assistant (LYNA) provides increases to both the speed at which pathologists can analyze slides and improved accuracy in detecting metastatic breast cancer within the slide samples used for the studies.
Google’s first study was published in the Archives of Pathology and Laboratory Medicine and investigated the accuracy of the algorithm using digital pathology slides. Google’s second study, published in The American Journal of Surgical Pathology, looked at how pathologists might harness the algorithm to improve workflows and use the tool in a clinical setting.
Medical laboratories and other diagnostics providers are already familiar with the improvement potential of automation and other technology-based approaches to diagnosis and analysis. Google’s LYNA is an example of how AI and machine learning improvements can serve as a supplement to—not a replacement for—the skills of experts at pathology groups and clinical laboratories.
Early research done by Google indicates that integrating LYNA into existing workflows could allow pathologists to spend less time analyzing slides for minute details. Instead, they could focus on other more challenging tasks while the AI analyzes gigapixels worth of slide data to highlight regions of concern in slides and samples for deeper manual inspection.
LYNA Achieves 99% Accuracy in Study of Metastatic Breast Cancer Detection
According to the research cited in a Google AI Blog post, roughly 25% of metastatic lymph node staging classifications would change if subjected to a second pathologic review. They further note that when faced with time constraints, detection sensitivity for small metastases on individual slides can be as low as 38%.
In findings published in Archives of Pathology and Laboratory Medicine, Google researchers analyzed whole slide images from hematoxylin-eosin-stained lymph nodes for 399 patients sourced from the Camelyon16 challenge dataset. Of those slides, researchers used 270 to train LYNA and the remaining 129 for analysis. They then compared the LYNA findings to those of an independent lab using a different scanner.
“LYNA achieved a slide-level area under the receiver operating characteristic (AUC) of 99% and a tumor-level sensitivity of 91% at one false positive per patient on the Camelyon16 evaluation dataset,” the researchers stated. “We also identified [two] ‘normal’ slides that contained micrometastases.”
Google’s algorithm later received an AUC of 99.6% on a secondary dataset.
“Artificial intelligence algorithms can exhaustively evaluate every tissue patch on a slide, achieving higher tumor-level sensitivity than, and comparable slide-level performance to, pathologists,” the researchers continued. “These techniques may improve the pathologist’s productivity and reduce the number of false negatives associated with morphologic detection of tumor cells.”
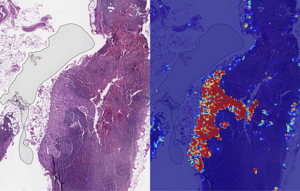
Left: sample view of a slide containing lymph nodes, with multiple artifacts: the dark zone on the left is an air bubble, the white streaks are cutting artifacts, the red hue across some regions are hemorrhagic (containing blood), the tissue is necrotic (decaying), and the processing quality was poor. Right: LYNA identifies the tumor region in the center (red), and correctly classifies the surrounding artifact-laden regions as non-tumor (blue). (Image and caption copyright: Google AI Blog.)
Faster Analysis through Software Assistance
Rapid diagnosis helps improve cancer outcomes. Yet, manually reviewing and analyzing complex digital slides is time-consuming. Time constraints might also lead to false negatives due to micrometastases or small suspicious regions that slip by pathologists undetected.
The Google research team of the study published in The American Journal of Surgical Pathology sought to gauge the impact LYNA might have on the histopathologic review of lymph nodes for trained pathologists. In their multi-reader multi-case study, researchers analyzed differences in both sensitivity of detecting micrometastases and the average review time per image using both computer-aided detection and unassisted detection for six pathologists across 70 slides.
Using the LYNA algorithm to identify and outline regions likely to contain tumors, the researchers found that sensitivity increased from 83% to 91%. The time to review slides also saw a significant reduction from 116 seconds in the unassisted mode to 61 seconds in the assisted mode—a time savings of roughly 47%.
“Although some pathologists in the unassisted mode were less sensitive than LYNA,” the researchers stated, “all pathologists performed better than the algorithm alone in regard to both sensitivity and specificity when reviewing images with assistance.”
The Future of Digital Pathology using LYNA
While the two studies show positive results, both studies also reveal shortcomings. Google highlighted both limited dataset sizes and simulated diagnostic workflows as potential concerns and areas on which to focus future studies.
Still, Google’s researchers believe that algorithms such as LYNA will help to power the future of diagnostics as healthcare in the digital era continues to mature. “We remain optimistic,” state the authors of the Google AI Blog post, “that carefully validated deep learning technologies and well-designed clinical tools can help improve both the accuracy and availability of pathologic diagnosis around the world.”
While other industries see risk in the growth of AI, both studies performed by researchers at Google show how computer-assisted workflows and machine learning could accentuate and bolster the skills of trained diagnosticians, such as anatomic pathologists and clinical laboratory technicians. By working to compensate for weak points in both human skill and computer reasoning, the outcome could be greater than either AI or humans can achieve separately.
—Jon Stone
Related Information:
Google Creates AI to Detect When Breast Cancer Spreads
Google Deep Learning Tool 99% Accurate at Breast Cancer Detection
Google’s AI Software Seeks to Detect Advanced Breast Cancer Better Than We Have Before
Google’s AI Is Better at Spotting Advanced Breast Cancer than Pathologists
Google AI Claims 99% Accuracy in Metastatic Breast Cancer Detection
Applying Deep Learning to Metastatic Breast Cancer Detection
Assisting Pathologists in Detecting Cancer with Deep Learning